Achieving Excellence in Machine Learning Assessments: Unraveling the 8 Key Qualities of Meaningful Homework
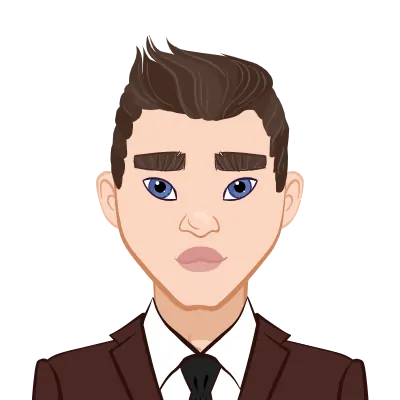
In today's technological environment, machine learning has developed into a potent tool that enables computers to learn from data and make wise decisions. Instructors and educators need to evaluate students' machine-learning knowledge and skills. Assessments must carefully take into account several essential factors to accurately assess students' knowledge and abilities. In this article, we'll examine the eight crucial characteristics that distinguish meaningful and successful machine-learning homework assessments from the rest. Educators can create tests that accurately gauge students' knowledge of and proficiency in machine learning by looking at these crucial factors, such as clear objectives, alignment with learning materials, real-world relevance, appropriate complexity, a balance between theory and practice, formative and summative elements, flexibility, and constructive feedback. Educators can design assessments that promote deep learning, critical thinking, and practical application skills in the field of machine learning by being aware of and incorporating these crucial characteristics. With the help of this article, educators will be better equipped to design assessments that will empower students and help them succeed in the dynamic field of machine learning.
.webp)
1. Clarity of Objectives and Expectations
To create meaningful assessments for machine learning homework, it is essential to make sure that the goals and expectations are clear. Teachers give students a clear roadmap of what is expected of them by outlining the assessment's objectives and outcomes. Students can focus their efforts more effectively on the assessment's purpose when there are clear objectives to guide them. Furthermore, clearly stated expectations lay out the precise standards by which students' performance will be judged. This clarity makes it possible for teachers to grade homework fairly and consistently while also assisting students in understanding what is expected of them. Students can effectively demonstrate their understanding and skills when they can align their learning efforts with the assessment process' clear objectives and expectations. Teachers can design tests that emphasize transparency, encourage student engagement, and allow for an accurate assessment of students' machine-learning capabilities by placing a strong emphasis on the definition of objectives and expectations.
Defined Learning Outcomes
Clear learning objectives must be established to create meaningful assessments. Effective assessment design is built on a clear definition of what students are expected to learn and accomplish through the machine learning homework. These learning objectives may be conceptual, practical, problem-solving-related, or any combination of these. Teachers give students a clear road map of what they need to do by clearly stating the desired learning outcomes. As a result of their understanding of the relevance and purpose of the assessments, students are better able to stay concentrated and motivated. Additionally, clear learning outcomes give teachers the ability to match course objectives with assessments, ensuring that the latter accurately reflects students' growth and achievement. The ability for students to track their progress and take charge of their learning journey is made possible by the communication of expectations to them when there are clear learning outcomes. Teachers can give students a meaningful and productive learning experience that equips them to succeed in machine learning by incorporating clearly defined learning outcomes into assessment design.
2. Alignment with Learning Materials and Instruction
Assuring alignment with learning materials and instruction is crucial when developing meaningful assessments for machine learning homework. Assessments ought to be closely related to the curriculum and teaching strategies employed during the entire learning process. Teachers give students a coherent learning experience by integrating assessments with the course materials, such as textbooks, lectures, and hands-on activities. This alignment makes it possible for students to apply their knowledge in a relevant setting while also reinforcing key concepts and understanding. Additionally, assessments that complement teaching strategies, like practical exercises or group projects, offer a consistent method of gauging students' learning advancement. Teachers make a seamless connection between what students have learned and what is required of them in assessments by integrating assessments with learning resources and instruction. By better reflecting students' understanding of machine learning concepts and their capacity to apply them successfully, this alignment increases the validity and reliability of the assessment.
Integration of Key Concepts
Aligning assessments with the learning resources and instructional content is essential to ensure that they accurately reflect the core concepts covered in the course. Assessments should gauge how well students comprehend and apply the fundamental ideas, formulas, and methods covered in the machine learning course. Teachers can assess students' comprehension of the fundamental knowledge and their capacity to apply it in real-world situations by incorporating key concepts into assessments. This alignment guarantees that tests accurately reflect students' understanding of key ideas and competence in applying machine learning tools and methodologies. Additionally, it emphasizes the relevance and usefulness of the learned knowledge by reiterating the relationship between theoretical concepts and their practical applications. By incorporating key concepts into assessments, educators can give students a thorough assessment of their learning outcomes, confirming both their understanding of the fundamental ideas and their aptitude for using machine learning techniques. This strategy improves the learning process overall and gets students ready for any difficulties they might run into in the machine-learning industry.
3. Authenticity and Real-World Relevance
Authenticity and real-world relevance are crucial when developing meaningful assessments for machine learning homework. Realistic tests replicate situations and difficulties that students might face in the field of machine learning. Authentic components, like real datasets, industry case studies, or simulated projects, give teachers a way to give students a chance to apply their knowledge and abilities in real-world situations. These tests not only look at students' theoretical knowledge but also at how well they can use machine learning to solve problems in the real world. Additionally, assessments that apply to real-world situations allow students to see the relevance and utility of what they have learned, which increases motivation and engagement. Educators promote a deeper understanding of machine learning concepts and their practical implications by aligning assessments with real-world scenarios. Additionally, genuine assessments foster analytical thinking, problem-solving abilities, and the capacity to modify machine-learning strategies for various contexts. Education professionals can help students develop the skills they need to work as competent, flexible machine-learning professionals by embracing authenticity and real-world relevance in assessments.
Application to Real-Life Scenarios
Many real-world applications heavily rely on machine learning. Enhancing students' comprehension and appreciation of the subject matter can be accomplished by designing assessments that mirror real-world situations. Authentic assessments give students the chance to put their knowledge and skills to use in solving real-world issues, helping them to grasp the relevance of machine learning in the real world. Teaching professionals can close the gap between theory and practice by creating assessments that reflect the difficulties and complexities encountered in actual machine learning projects. These evaluations might entail studying actual datasets, creating predictive models for particular industries, or using machine learning algorithms to solve challenging issues. Students who participate in authentic assessments gain useful experience using machine learning strategies to address real-world issues, preparing them for future careers. Students are also better able to comprehend the impact and potential of machine learning in a variety of industries, including transportation, finance, and healthcare. A meaningful learning experience that connects theory and practices is created by educators by incorporating real-world situations into assessments, giving students the knowledge and skills they need to succeed in the fast-paced field of machine learning.
4. Complexity and Challenge
Including the right amount of complexity and challenge when creating meaningful assessments for machine learning homework is essential. Assessments should encourage students to think critically and solve problems, rather than just test their ability to recall information. Teachers can evaluate their student's ability to apply machine learning principles in intricate situations by giving them challenging tasks. Students should be required to analyze, interpret, and manipulate data as well as to make defensible decisions based on their comprehension of machine learning algorithms and techniques in these assessments. Assessments encourage students' intellectual development by embracing complexity and challenge, fostering their capacity to address actual machine-learning issues. Furthermore, difficult assessments encourage persistence, resiliency, and creativity while letting students push the limits of their knowledge and abilities. Educators can accurately assess students' depth of understanding and ability to solve challenging machine-learning problems by giving assessments that correspond to the complexity of the subject matter.
Gradation of Difficulty
Student skill levels, from novice to expert, should be catered for ineffective machine learning assessments. Instructors can push students to apply their knowledge in more challenging situations by incorporating questions or problems of varying complexity. This grading of difficulty makes sure that tests are properly adapted to students' skills and promotes their development. Students can demonstrate their proficiency and problem-solving abilities at various stages of their learning journey by taking assessments with a variety of difficulty levels. Beginners can demonstrate their fundamental knowledge, while more experienced students can be tested with more difficult and sophisticated tasks. As a result of having to evaluate and apply their knowledge in a variety of contexts, students are encouraged to develop their critical thinking and problem-solving abilities. Gradually raising the bar on assessments fosters a sense of accomplishment and motivation in students by continuously pushing them to broaden their machine-learning knowledge and skill set. Instructors foster a supportive and demanding learning environment that encourages continuous improvement and equips students for problems with machine learning in the real world by grading assessments' difficulty.
5. Balance between Theory and Practice
Writing effective assessments for machine learning homework requires striking a balance between theory and practice. The assessment of machine learning concepts should cover both theoretical understanding and real-world application. Teachers can make sure that assessments reflect real-world situations while also gauging how well their students understand fundamental concepts by balancing theory and practice. This balance enables students to show that they can apply abstract ideas to concrete situations, strengthening their understanding and problem-solving abilities. Students get hands-on experience and the chance to hone their skills when assessments include practical elements like coding challenges, data analysis tasks, or model implementation. Additionally, assessments that incorporate theoretical queries or conceptual comprehension guarantee a thorough assessment of students' theoretical knowledge. To assess students' ability to bridge the gap between theoretical understanding and practical application, educators must strike a balance between theory and practice. This balance will help students succeed in real-world machine-learning scenarios.
Theoretical Understanding and Hands-On Implementation
The field of machine learning necessitates both theoretical comprehension and actual application. Assessments should balance testing conceptual understanding with gauging one's capacity to use machine learning models and algorithms. Teachers can provide a thorough assessment of students' overall competence by incorporating both theoretical questions and practical coding exercises. Assessments of theoretical understanding gauge students' understanding of important machine learning concepts, tenets, and methodologies. They evaluate their capacity to decipher results, interpret algorithms, and assess the influence of various parameters. On the other hand, practical implementation assessments test students' ability to use machine learning methods on datasets from the real world. These tests evaluate a person's capacity for data preprocessing, model construction and training, performance optimization, and result interpretation. To ensure that students have a well-rounded proficiency in machine learning, educators combine theoretical knowledge with practical application assessments. With this method, students can demonstrate their theoretical and practical knowledge and skills, getting them ready for the demands and challenges of the field.
6. Formative and Summative Assessment Elements
It is crucial to include both formative and summative assessment components when creating meaningful assessments for machine learning homework. Students can continuously monitor their progress, pinpoint areas for improvement, and modify their learning strategies by using formative assessments, which offer ongoing feedback and opportunities. These evaluations, which typically have low stakes, are meant to support students' learning and skill growth. Summative tests, on the other hand, assess students' overall knowledge and competence after a unit, course, or academic year. They usually have high stakes and offer a general assessment of students' performance. To create a thorough evaluation process, educators can combine elements of formative and summative assessment. Summative assessments offer a final assessment of students' accomplishments while formative assessments allow students to consider their learning process. This combination offers a comprehensive view of students' learning outcomes in addition to encouraging continuous improvement. Machine learning homework assessments that include both formative and summative assessment components improve students' learning and allow teachers to give them individualized feedback and support for their development.
Continuous Feedback and Final Evaluation
Evaluations can be used for summative and formative purposes. Students receive continuous feedback from formative assessments, enabling them to pinpoint their areas of weakness and track their development. Students are kept engaged and motivated while developing a growth mindset thanks to this ongoing feedback. Summative tests, on the other hand, assess students' overall performance and establish their level of mastery. These final assessments offer a thorough snapshot of the machine-learning skills of the students at a particular time. To create a balanced and comprehensive evaluation process, educators combine both formative and summative assessment components. Summative assessments offer a conclusive assessment of students' knowledge and skills, while formative assessments assist students on their learning journey. This combination enables teachers to give students the support and direction they need while also assessing their overall machine-learning performance. Together, the ongoing feedback and the final assessment help to create an evaluation system that encourages learning supports student growth and gives a reliable indication of how well-versed a student is in machine learning.
7. Flexibility and Adaptability
Flexibility and adaptability must be included in assessments for machine learning homework to be meaningful. Assessments should be created in a way that accommodates individual variations in learning preferences, pace, and styles. To accommodate different student needs and foster an inclusive learning environment, educators offer flexibility, such as various assessment formats or alternative approaches. Flexibility also enables adaptation to unforeseen events or modifications in the educational environment. Assessments should be flexible enough to accommodate new developments in machine learning, emerging trends, and technology. To maintain assessments' relevance and accurately gauge students' proficiency in the newest tools and techniques, educators must stay up-to-date and responsive to these changes. The inclusion of flexibility and adaptability in assessments encourages students to explore and apply machine learning concepts in ways that are consistent with their interests and objectives and empower them to take control of their learning journeys. In the end, adaptability and flexibility in assessments encourage student engagement, drive, and a sense of ownership over their educational journey.
Multiple Assessment Methods
Not all students acquire knowledge or demonstrate it in the same way. Incorporating a variety of assessment techniques, such as written exams, coding homework, or presentations, allows students to demonstrate their strengths while accommodating different learning styles. Giving students options for assessment formats encourages inclusivity and gives students' machine-learning skills a more accurate representation. By utilizing a variety of assessment techniques, teachers can assess students' knowledge and abilities thoroughly by drawing on their various cognitive capacities and skills. Along with encouraging creativity and critical thinking, this strategy helps students navigate various assessment tasks. Additionally, it gives students the chance to develop and hone a variety of machine learning-related skills, including communication, coding, and problem-solving. Teachers can foster a culture that values individual talents and encourages students' all-around development in the field of machine learning by embracing a variety of assessment techniques.
8. Constructive Feedback and Learning Opportunities
Making meaningful assessments for machine learning homework requires providing constructive criticism and providing learning opportunities. Assessments ought to offer students actionable feedback that directs their learning and development in addition to evaluating their performance. Constructive criticism identifies areas of strength and weaknesses and offers detailed suggestions for improvement. It enables students to focus their efforts on areas that need improvement by assisting them in understanding their strengths and weaknesses. Assessments should also include learning opportunities that let students put the criticism they get to use and hone their abilities. These changes might come in the form of extra practice tasks, revision homework, or group discussions to help them understand concepts better. Exams become transformative experiences that aid students' ongoing learning by incorporating helpful criticism and learning opportunities. They promote introspection, evaluation of oneself, and the growth of metacognitive abilities. Students are given the tools to become active participants in their learning process through constructive feedback and learning opportunities, which promotes a growth mindset and enables them to continuously advance their machine-learning skills.
Detailed Assessment Feedback
Meaningful assessments give students helpful feedback rather than just a grade. Students benefit from detailed assessment feedback, which identifies areas of strength and those that require development. With the help of this feedback, students can track their development, make the necessary corrections, and better understand and practice machine learning. Assessments can also be turned into learning opportunities by offering resources or advice for additional study, which encourages continuous improvement. By providing detailed instructions, suggested readings, or online tutorials, educators enable students to pursue independent learning and broaden their knowledge beyond what is required for the assessment. With the help of this method, students are given the tools they need to succeed in their machine-learning endeavors and are encouraged to adopt a lifelong learning mindset. Students can strive for excellence and realize their full potential in machine learning by working in a supportive and enriching environment that combines detailed assessment feedback with additional learning resources.
Conclusion
Making meaningful assessments for students' machine learning homework is crucial for advancing their knowledge of and competence in this quickly developing field. Teachers can create tests that accurately assess students' knowledge and skills by incorporating the eight essential characteristics covered in this article: clear objectives, alignment with learning materials, real-world relevance, appropriate complexity, a balance between theory and practice, formative and summative elements, flexibility, and constructive feedback. These tests encourage critical thinking, problem-solving skills, and the practical application of machine learning concepts in addition to rote memorization. Assessments transform into effective tools for continuous improvement by offering insightful feedback and fostering growth opportunities. Educators must modify assessments to reflect new trends and technologies as machine learning continues to change industries. In the end, educators can equip students to thrive in the dynamic world of machine learning and position them for success in this exciting field by designing meaningful assessments.